In our previous blog, we discussed the basics of bio-ontologies. Now, we're diving deeper to understand the challenges posed by non-standardized vocabularies and exploring the emerging need for customized ontologies.
Challenges of Non-standardized Vocabulary
The development of bio-ontologies has evolved through numerous independent efforts, each contributing to the broad diversity seen in the field.
The diversity seen in the field of bio-ontologies presents challenges in achieving interoperability due to overlapping terminologies and distinct conceptual frameworks across ontologies.
Initiatives often start without direct coordination, reflecting the wide-ranging goals and applications in the field—from genomic data annotation to phenotypic variability and biological pathway modeling. This diversity presents challenges in achieving interoperability due to overlapping terminologies and distinct conceptual frameworks across ontologies.
Here are the main drawbacks of using non-standardized vocabulary:
► Inconsistency:
Often, the use of standardized terminology in biology and the life sciences proves inadequate. Varied terminologies and classifications can lead to confusion and inconsistencies in data interpretation and analysis. Additionally, these discrepancies can cause issues in data retrieval, resulting in missing or skewed data.
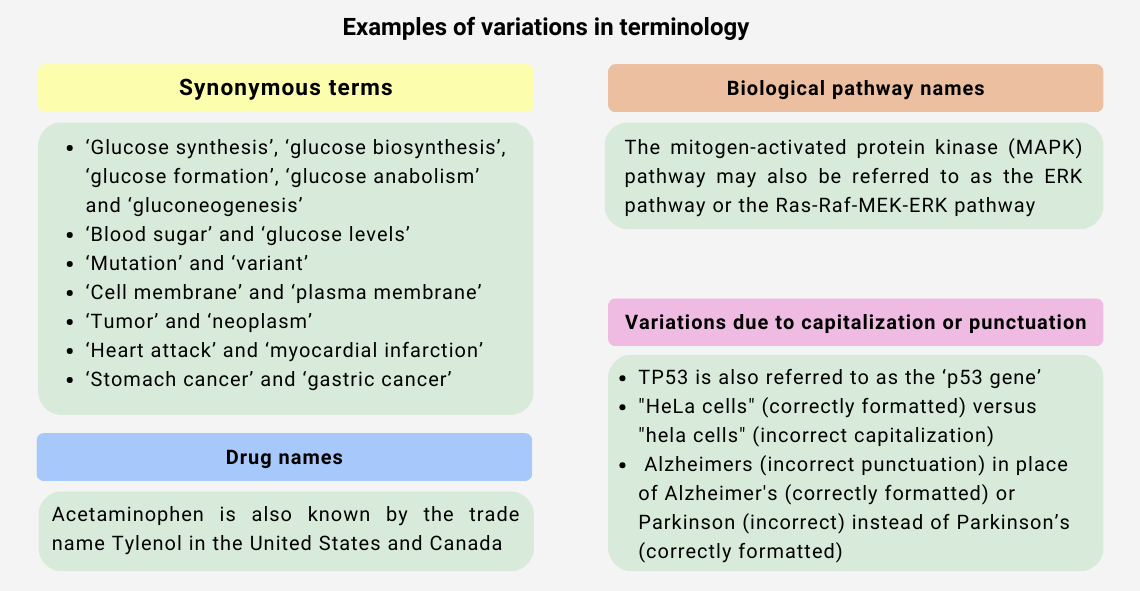
► Data fragmentation and limited discoverability:
These challenges arise from two main issues:
- The absence of standardized metadata complicates the efficient search and retrieval of specific data.
- The prevalence of heterogeneous data formats and terminologies hinders effective data integration and sharing.
► Impaired decision-making and obstacles in collaborative research:
Informed decision-making is hindered by inconsistencies and challenges in integration, which complicate the thorough analysis of comprehensive data. For example, when data from different sources cannot be easily combined, it limits the ability to draw accurate conclusions.
Without standardized data organization, managing information becomes inefficient, creating barriers to collaboration and knowledge sharing. Vital data often gets trapped within specific departments, restricting wider access. Moreover, the extra effort needed to prepare and standardize data for cross-dataset searches adds significant time and resources for researchers.
When data from different sources cannot be easily combined, it limits the ability to draw accurate conclusions.
Requirement for Controlled Vocabulary
A controlled vocabulary employs precise, universally recognized terms to define and categorize concepts within specific fields. As highlighted in the examples above, the fields of biology and life sciences at large are replete with synonyms, abbreviations, and acronyms for the same concept. Ontologies streamline this by assigning unique identifiers to each entity and recording alternate names with metadata, thus ensuring consistent terminology in describing biomedical entities, their functions, and disease associations.
The Emerging Need for Customized Ontologies
Customized ontologies are tailored frameworks designed to organize and interpret biological and medical information according to the unique needs and specifications of specific clients or organizations.
Aligning an ontology’s structure and terminology with an organization's unique research objectives, data types, and workflows can significantly enhance data management, analysis, and decision-making processes.
In some instances, publicly available ontologies may not offer adequate coverage for specific applications, or they might require refinement to capture nuances absent in standard ontologies. Aligning an ontology’s structure and terminology with an organization's unique research objectives, data types, and workflows can significantly enhance data management, analysis, and decision-making processes.
At Strand, we provide customized ontology solutions for organizations aiming to develop domain-specific vocabularies. One of our clients, a leading pharmaceutical company, sought to create an ontology tailored to their research on single-cell data. Similarly, another client, a biotech firm, aimed to develop an ontology focused on specific areas of the heart.
Strand successfully developed customized ontologies for both clients by leveraging standard databases, as well as our proprietary database, further enriched by curating data from research publications specified by the client. Our database serves as an excellent starting point for clinical and 'omics' data, especially single-cell omics data.
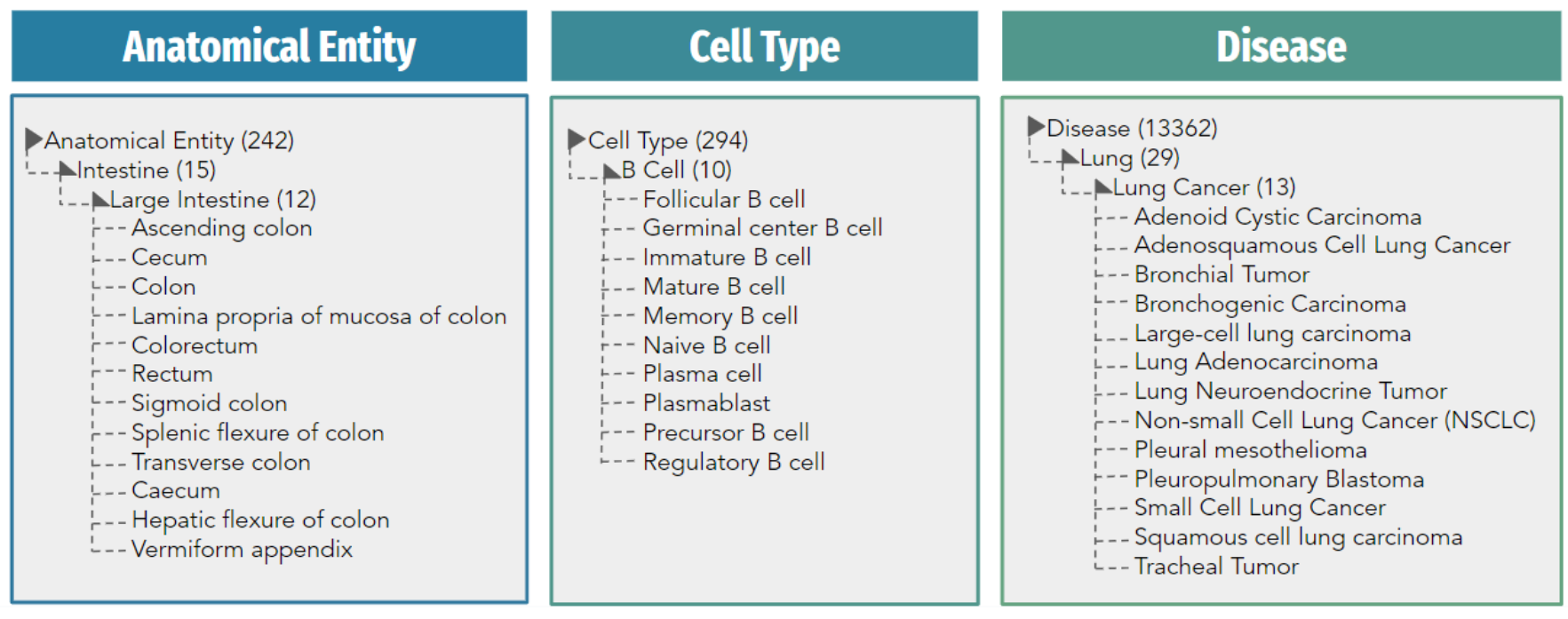
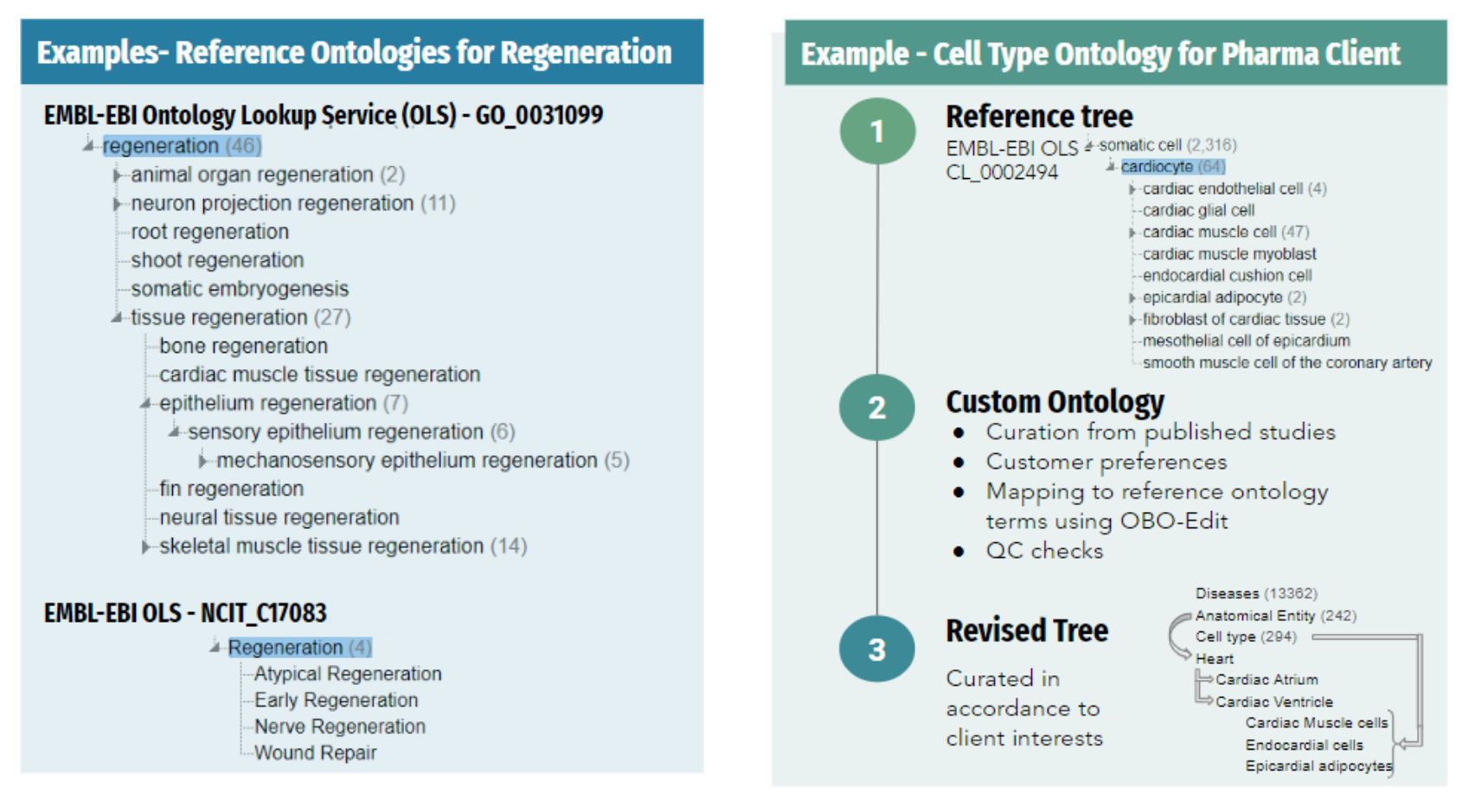
Wrapping up, it's evident that as biology leans more into the data realm, the necessity for systematic description of existing biological knowledge can't be overstated. The challenges associated with the absence of such systems underscore the importance of adopting ontology databases. They're the key to sorting out data, ensuring we can access and utilize the information we need effortlessly.
For a more in-depth exploration of how we helped a big pharmaceutical company with data harmonization, read this case study!
You can find the rest of the blog series below:
- Part 1: From Chaos to Clarity: Harmonizing Data
- Part 2: What’s in a Term?
- Part 4: Harnessing the Power of Harmonized Data: Strand’s Approach
References:
- Biological and Medical Ontologies: Introduction, Marco Masseroli, in Encyclopedia of Bioinformatics and Computational Biology, 2019
- Jensen LJ, Bork P. Ontologies in quantitative biology: a basis for comparison, integration, and discovery. PLoS Biol. 2010 May 25;8(5):e1000374. doi: 10.1371/journal.pbio.1000374. PMID: 20520843; PMCID: PMC2876043.
- Leonelli S. Documenting the emergence of bio-ontologies: or, why researching bioinformatics requires HPSSB. Hist Philos Life Sci. 2010;32(1):105-25. PMID: 20848809.
- Bodenreider O, Stevens R. Bio-ontologies: current trends and future directions. Brief Bioinform. 2006 Sep;7(3):256-74. doi: 10.1093/bib/bbl027. Epub 2006 Aug 9. PMID: 16899495; PMCID: PMC1847325.