Biomedical insights based on a single omics level are only one piece of the puzzle.
Integrating and analysing various omics layers—genomics, transcriptomics, proteomics, metabolomics and others—allows us to probe further into disease pathways and potential drug targets.
With these multi-layered molecular insights, we are able to predict disease, accelerate drug discovery and provide personalized treatments to patients.
However, bringing high-throughput multi-omics data together comes with the challenges of data integration and management.
Combining diverse datasets is not as straightforward because of the complexities present in individual datasets. Often, there are missing values, inconsistent metadata, and non-standard naming methods.
This was brought to light at a recent talk I was at as part of Bio-IT World 2024, where Dr Joseph Pearson, Global Product Manager, Qiagen, elaborated on the benefits of unified omics data and how it can accelerate bioinformatic prioritization of drug targets. He mentioned that data scientists and bioinformaticians spend 80% of their time cleaning data because it is important to have integrated data for answering biological questions.
Thus, data integration - including onboarding, cleaning, harmonizing and manual curating - is a crucial step on our path toward multiomics discovery.
On this note, we presented a poster at Bio-IT World 2024 emphasizing our strategies for managing multi-omics datasets through harmonization and curation.
It is becoming increasingly clear that good data management cannot happen in siloes. This is especially true for multi-omics data, which needs various experts to understand and process the breadth of information. At Strand, curators, bioinformaticians, data scientists, and software engineers work together to make this happen.
Curators, bioinformaticians, data scientists and software engineers work together to manage the workflow from data ingestion to uploading of harmonized data for user access.
The process begins with data ingestion: we onboard multi-omics data from various sources such as public databases (GEO, SRA and ENA), publications, cloud-based data repositories, custom databases and labs and integrate it into a unified format.
Next, we apply various harmonization and curation methods to prepare them for downstream sharing and analysis. These include:
- using controlled vocabulary
- developing metadata schemas
- enforcing naming conventions
- providing clear definitions
Finally, when the datasets are harmonized, we upload them to a cloud or any other specified location with appropriate access controls.
This streamlined system has enabled us to:
- harmonize over 1000 datasets to improve gene models
- source scRNA and scATACdata for model building
- create an integrated atlas and a time-series model for cell-cell interaction studies
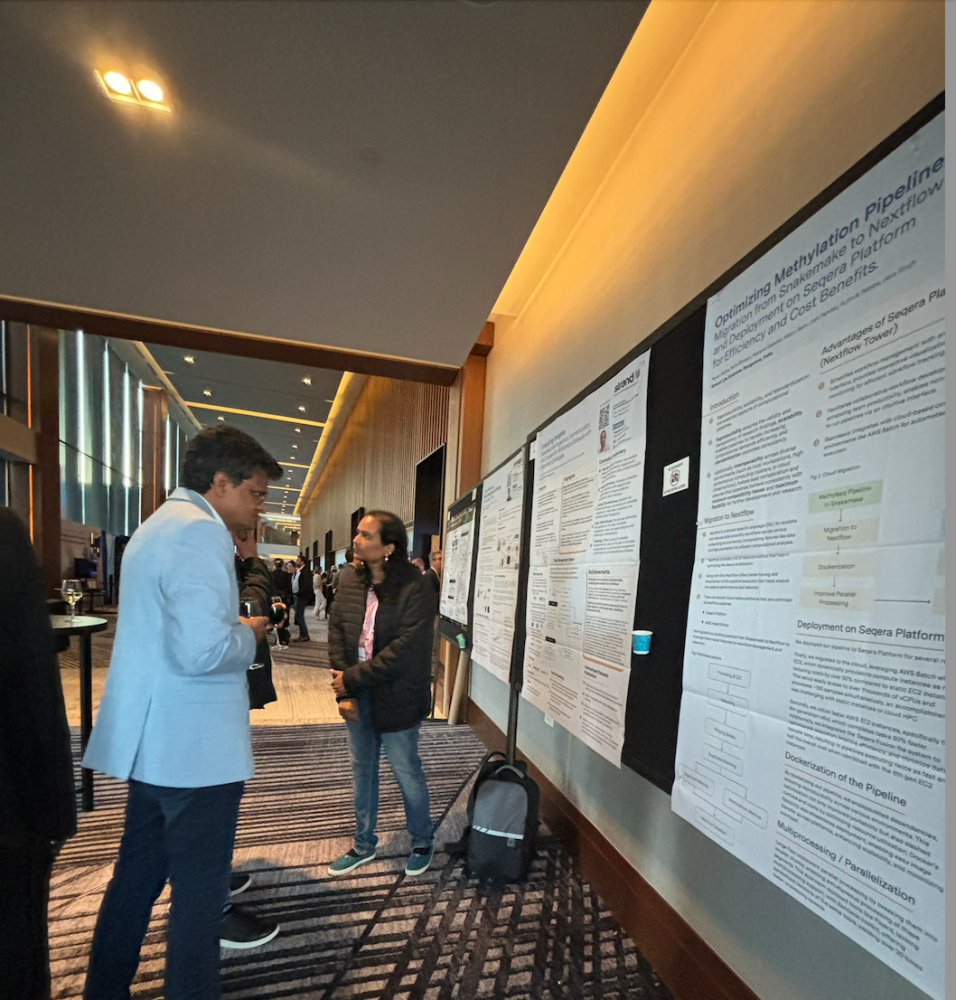
Our efforts in standardizing and managing diverse datasets do not end here. We’re actively working on improving and automating the metadata curation process. For this, the team is experimenting with LLM automation for ontology mappings.
You can download a PDF version of this poster from our website!
Feel free to get in touch with Yasodha Kannan Sivasamy Radhakrishna Bettadapura Jaya Singh, PhD Ernie Hobbs to learn about our data harmonization services.