Spatial imaging is a key technology in biomedical research, allowing researchers to visualize cellular structures and their interactions in their natural settings. By combining it with spatial transcriptomics, researchers can better understand cell and tissue architecture and gene expression patterns.
What is Cell Segmentation?
Cell segmentation, a fundamental computational technique, distinguishes and defines individual cell boundaries within biomedical images. This process is vital for extracting important biological information from images, aiding researchers in understanding cellular structures and dynamics in detail.
Why is Cell Segmentation Important?
Cell segmentation plays a crucial role in bioimage informatics, forming the basis for various detailed studies. Its primary goal is to approximate the boundaries between cells, enabling the accurate assignment of transcripts to individual cells. Here’s how it helps:
- Cell Counting: It quantifies the cells in a sample, crucial for various biological assessments.
- Morphological Analysis: Researchers can examine the shape, size, and structure of cells, gaining insights into their functional potentials.
- Cell Tracking: This involves monitoring cell movements and behaviors over time, essential for dynamic biological studies.
- Response Assessment: It aids in assessing how cells respond to treatments or changes in their environment.
Inaccuracies in segmentation can introduce significant errors, compromising the integrity of research findings.
Methods of Cell Segmentation
There are two main approaches to cell segmentation:
1. Traditional Techniques: These methods rely on the intensity and gradient of images to differentiate cells from their surroundings.
- Thresholding: This method sets a specific intensity value as a threshold to separate cells from the background, classifying pixels above this threshold as cells.
- Watershed Transformation: Here, the image is treated as a topographic map with varying elevations, where the light intensities represent these elevations. The image is segmented into distinct regions based on these topological variations, effectively isolating overlapping cells.
2. Deep Learning Approaches: The advent of artificial intelligence has popularized deep learning techniques, such as convolutional neural networks (CNNs) and models like Mask R-CNN. These methods automatically learn from large datasets and are especially good at segmenting complex, overlapping cells. For instance, tools like Cellpose and DeepCell have been recognized for their effectiveness in various experimental settings.
Evaluating Segmentation Methods
Choosing the right segmentation method involves evaluating their performance across various metrics, such as accuracy, adaptability to different imaging modalities, and efficiency with various cell types. Recent studies have shown that deep learning methods generally offer superior performance over traditional techniques, particularly when trained on diverse and large datasets.
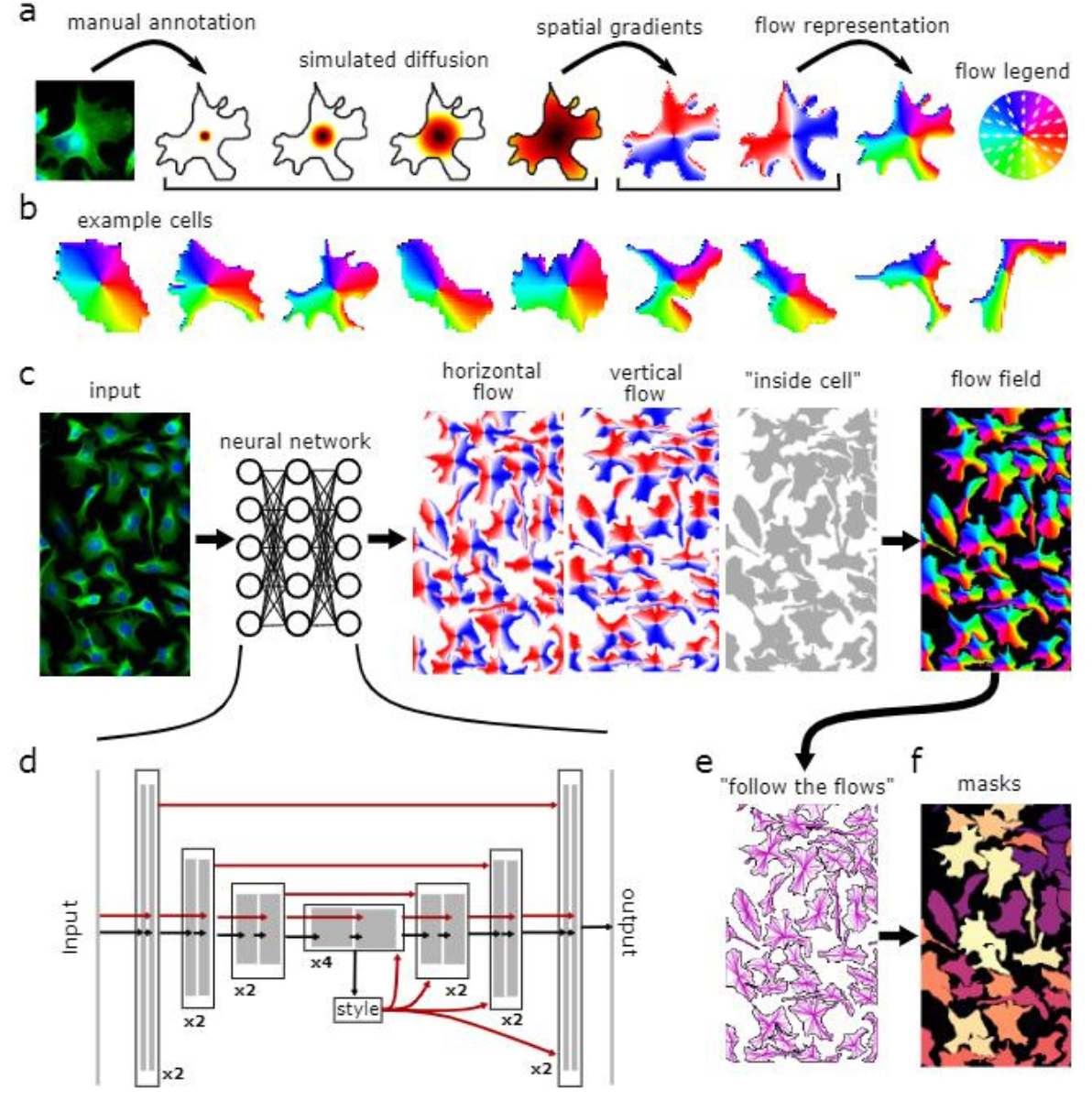
Figure 1: Model Architecture: a, Converting annotated masks into vector flows for neural network analysis using a diffusion process to point gradients toward cell centers. b, Training data examples of spatial gradients. c, Neural network predicts gradients and pixel cell membership, merging these into a vector field. d, Neural network with a U-Net3 backbone processes feature maps and integrates skip connections. e, At testing, predicted vector fields help form dynamic systems to define masks. f, Pixels converging to the same point are classified under one mask.
In a recent in-house analysis, the Strand pipeline, equipped with advanced Cellpose3 technology, excelled in cell segmentation. It captured more cells, including those previously missed by another pipeline (figures 2-4), and precisely identified cells in critical regions. Cells with very low transcript levels were effectively filtered out to maintain data quality. These results highlight the Strand pipeline's accuracy and effectiveness in supporting detailed cellular studies.
Comparative Analysis Results
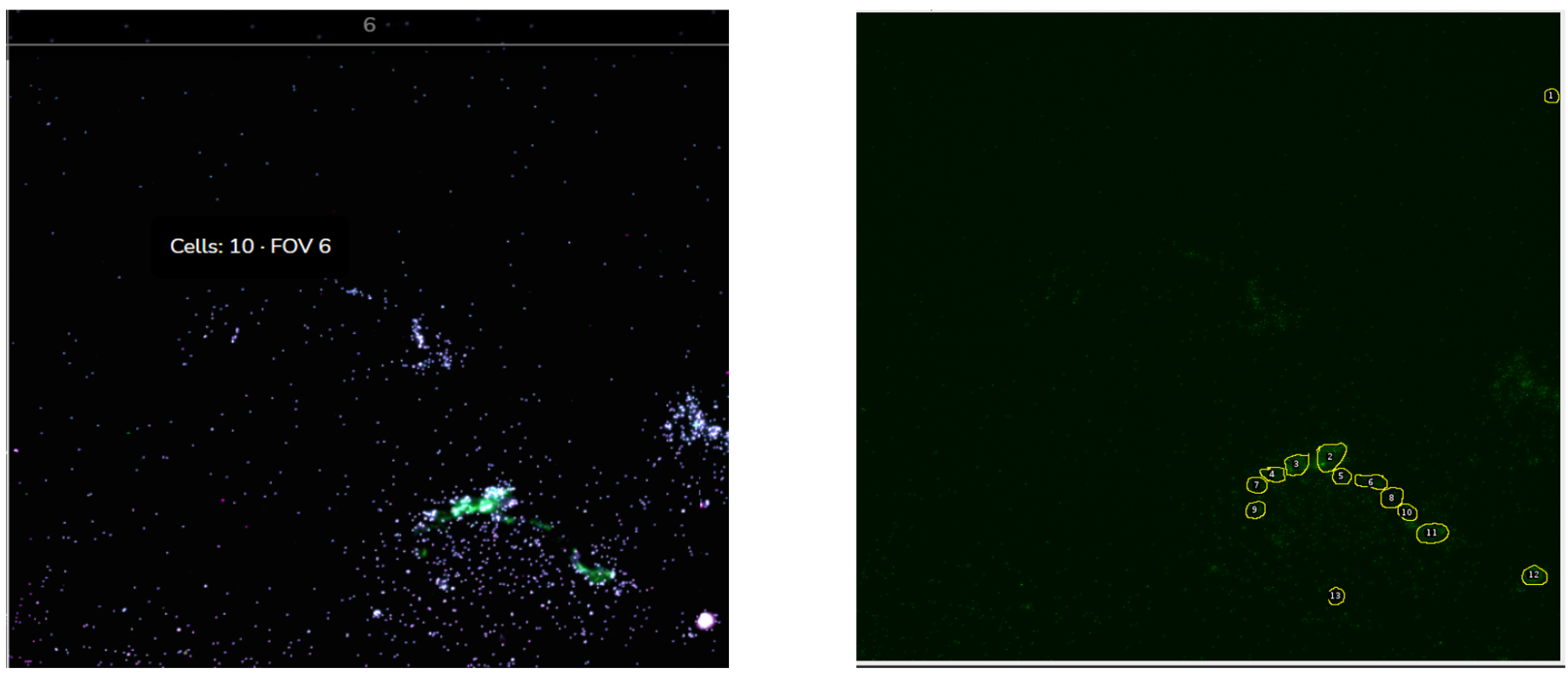
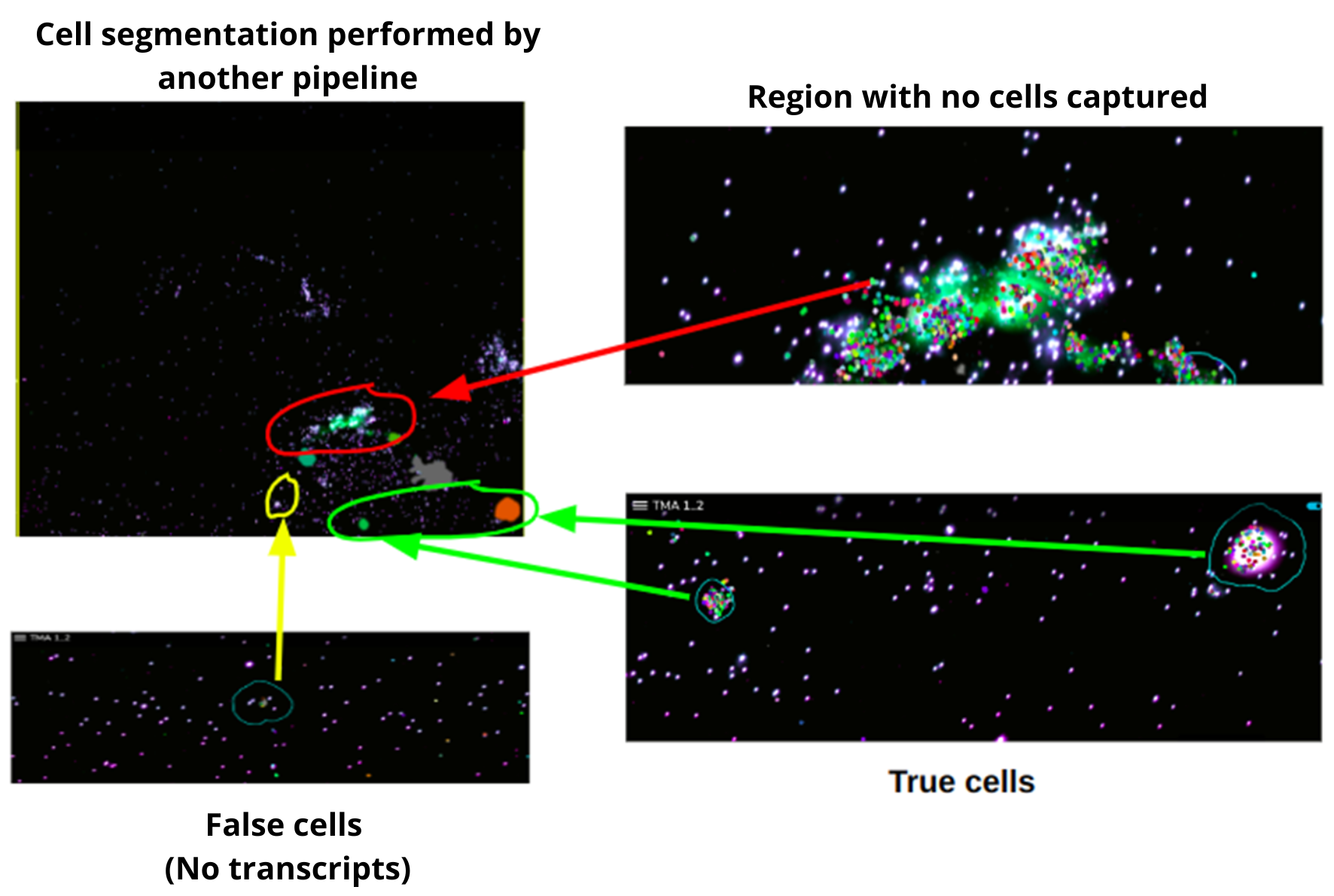
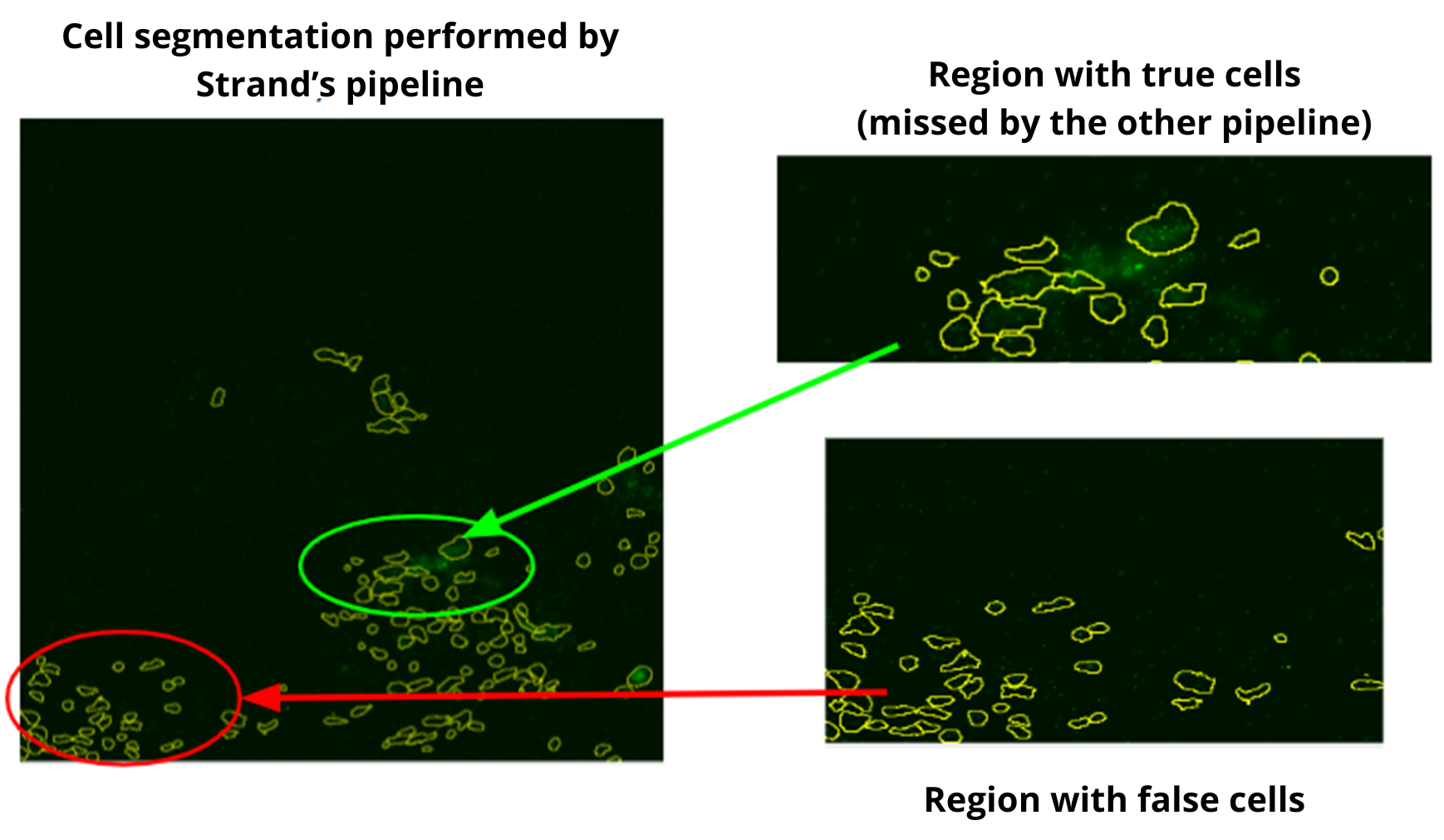
At Strand, we employ our in-house segmentation pipeline which is based on Cellpose 3.0, for spatial imaging. This pipeline excels in detecting and analyzing cells within complex images. We have rigorously trained our model on a diverse range of images to ensure it captures a high number of cells accurately, even in typically challenging areas, allowing for precise analysis of transcription levels. This accuracy is crucial for in-depth cellular studies and enhances our potential to drive significant advancements in cellular research.
Banner Image courtesy Cellpose: Deep Learning-Based, Generic Cell Segmentation - 2021- Wiley Analytical Science
References:
- Chen H, Murphy RF. Evaluation of cell segmentation methods without reference segmentations. Mol Biol Cell. 2023 May 15;34(6):ar50. doi: 10.1091/mbc.E22-08-0364.
- Li Y, Lac L, Liu Q, Hu P. ST-CellSeg: Cell segmentation for imaging-based spatial transcriptomics using multi-scale manifold learning. PLoS Comput Biol. 2024 Jun 27;20(6):e1012254. doi: 10.1371/journal.pcbi.1012254. PMID: 38935799; PMCID: PMC11236102.