Real-World Data
Real-World Data
Bridging the Gap Between RWD and Powerful Clinical Insights
Our Expertise in Handling RWD Data Can Help You
Our Expertise in Handling RWD Data Can Help You
01
Derive Real-World Insights from Curated Datasets
We have built discovery cohorts in multiple areas of disease (oncology & non-oncology) and demonstrated real-world insights with curated datasets such as AACR GENIE in NSCLC.
02
Enable Precision Analytics for Patient Stratification
Our in-depth mutation-based and stage-specific stratification can power precision medicine using RWD, including assessment of immunotherapy outcome. We were recently approached by a biopharmaceutical company looking to leverage public datasets for improved patient stratification based on drug response biomarkers in ulcerative colitis. Through a proprietary workflow, we were able to analyze ~ 3000 samples, identifying five markers with 100% sensitivity at 70% specificity. This involved ingesting both public and client datasets, integrating literature-derived markers and machine learning-based response prediction, and creating a Disease Activity Score.
03
Leverage a Robust Data Harmonization Framework
We harmonize varied datasets in a documented process, providing format uniformity, ontology consistency, and analysis preparedness at scale. The raw data we often work with varies in terms of data formats (FASTQ, BAM, CSV, etc), experiment types (Microarray, RNA-seq, ChiP-Seq, single-cell sequencing, etc), and cross-species data, among many other attributes. The harmonization process involves integrating and standardizing this heterogeneous data to ensure consistency, compatibility, and accuracy across datasets.
04
Accelerate and Scale Data Curation and Harmonization through LLMs
GPT-based tools drive acceleration of data ingestion, annotation, and curation at 95 % + accuracy, minimizing manual effort and turnaround time by 3x. In-house tool festiVAR supports scalable interpretation of rare disease with ACMG scoring and gene-phenotype correlation by LLM.
Our Offerings in Real World Data Studies and Harmonization
Our Offerings in Real World Data Studies and Harmonization
- Strand has deep expertise in working with clinical omics data, and we are also establishing our own in-house RWD database, owing to our 24+ years of experience developing genomics solutions. We leverage this background for analyzing any type of RWD datasets.
- We have recently conducted two research studies using the AACR project Genie NSCLC datasets. Read more about it in this white paper.
Read more about it in this white paper
- If data has been obtained from various sources (EHRs, claims data) & multiple providers, we standardize all the data and then harmonize it using a unified data model and create data pipelines. This enables downstream analysis of harmonized data.
- Strand has been developing an in-house RWD data repository by collecting clinical data from various sources. We apply the following workflow to harmonize this data. Below is an example of a workflow we have implemented to analyze the in-house RWD data. This process can be customized as required.
- A solution to unify in-house clinical data into standardized, analyzable format
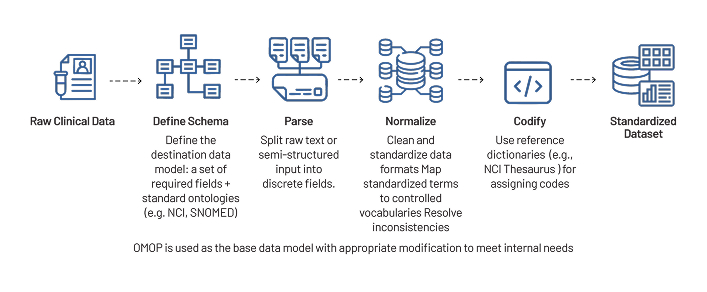
RWD Studies
- Strand has deep expertise in working with clinical omics data, and we are also establishing our own in-house RWD database, owing to our 24+ years of experience developing genomics solutions. We leverage this background for analyzing any type of RWD datasets.
- We have recently conducted two research studies using the AACR project Genie NSCLC datasets. Read more about it in this white paper.
Read more about it in this white paper
Harmonizing
RWD Before
Analysis
- If data has been obtained from various sources (EHRs, claims data) & multiple providers, we standardize all the data and then harmonize it using a unified data model and create data pipelines. This enables downstream analysis of harmonized data.
- Strand has been developing an in-house RWD data repository by collecting clinical data from various sources. We apply the following workflow to harmonize this data. Below is an example of a workflow we have implemented to analyze the in-house RWD data. This process can be customized as required.
- A solution to unify in-house clinical data into standardized, analyzable format
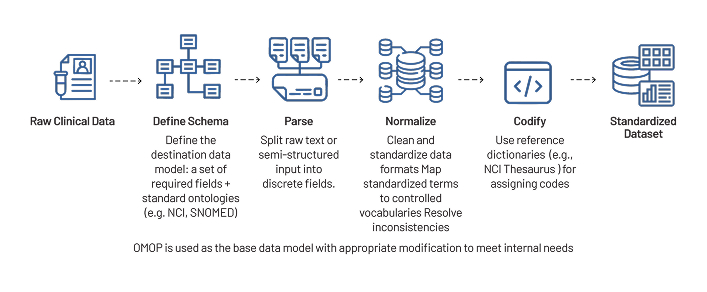
Frequently Asked Questions
Frequently Asked Questions
Here is a quick summary of the data heterogeneity we work with regularly.